Introduction to AI in Healthcare Technology Submissions
In recent years, artificial intelligence (AI) has transformed various industries, from healthcare to finance, with its ability to analyse vast amounts of data and extract valuable insights. For example, AI has been used to read and assess financial documents and reports, and extract data from them [1]. It can also learn rules, perform auditing, pick up on non-compliant content to reduce reimbursement errors and waste [2]. Algorithms are often making recommendations for what shares to buy or sell based on large-scale automated machine-learning computational processes “that identify positive, negative, and neutral opinions” from news and public reports and disclosures [3]. In the financial sector, some organizations have started to tailor their reports based on “machine readability” by using or not using words that would be interpreted as positive or negative, respectively, by an algorithm in order to generate a favourable outcome and conclusion about the content [1]. In other words, humans are changing their behaviour, writing style, and content used in order for their work to be perceived positively by AI.
Could and should AI be used to develop documents for regulatory approval and health technology assessments?
Health technologies such as medications and medical devices are subject to regulatory approval by regulatory agencies such as the European Medicines Agency (EMA). Once a medication or medical device is approved, it undergoes a formal Health Technology Assessment (HTA) to take its efficacy and cost (amongst others) into consideration and advise decision-makers on how to best prioritize and efficiently allocate limited resources [4,5]. Although not required in all countries, many countries use HTAs to decide whether to use public funds to pay for expensive new health technologies [4]. HTAs are also used by doctors and patients to make decisions about treatments, and by researchers to identify lack of knowledge or gaps in evidence [4]. HTA agencies assess medications from an economic, ethical and social perspective and take budget impact, comparative-effectiveness, and cost-effectiveness into consideration in order to make a decision about reimbursement [5]. HTA agencies and manufacturers, with the help of health economic and outcomes research (HEOR) companies, often develop dossiers to support decision-making and improve market access [5,6]. These dossiers can be hundreds of pages in length, and writing and reviewing them is very labour intensive. Dossier development and HTAs rely on systematic literature reviews (SLR) to find the most relevant and best available evidence to answer certain questions for appropriate decision-making [5,7].
In this blog, we'll explore how AI is revolutionizing the process of crafting regulatory submissions and Global Value Dossiers (GVDs), and the benefits it brings to stakeholders in the healthcare ecosystem.
Understanding Regulatory Submissions and GVDs
Before diving into the role of AI, let's first understand what regulatory submissions and GVD entail.
Regulatory submissions are documents submitted to regulatory agencies, such as the U.S. Food and Drug Administration (FDA) or the European Medicines Agency (EMA), to seek approval for the marketing and sale of pharmaceutical products or medical devices. These submissions include extensive scientific data, clinical trial results, safety profiles, and other information necessary for regulatory approval.
GVDs are comprehensive documents that provide evidence-based assessments of the value of a pharmaceutical product or medical device. These dossiers are essential for demonstrating the clinical and economic benefits of a product to payers, healthcare providers, and regulatory agencies worldwide.
How AI Can Enhance Regulatory Submissions and GVDs
AI is revolutionizing the process of crafting regulatory submissions and GVDs in several ways:
Data Extraction, Analysis and Synthesis
AI algorithms can extract relevant information from clinical trials, real-world data, and scientific literature. AI-powered algorithms can analyse large datasets of clinical trial data, real-world evidence, and economic models to extract relevant information and insights. This data analysis helps in synthesizing evidence to support the value proposition of a product in GVDs and regulatory submissions.
Machine learning (ML)
ML algorithms (which is part of AI) have been used to screen titles and abstracts during SLRs, and performed relatively well in terms of precision, sensitivity and specificity.7,8 Well-performing algorithms can cut down substantially on the time and people needed to perform SLRs.9 This can accelerate the evidence-gathering process for GVDs. Machine learning models can also predict treatment effects, cost-effectiveness, and patient-reported outcomes.
Natural Language Processing (NLP)
NLP algorithms enable AI to parse through vast amounts of scientific literature, regulatory guidelines, and medical databases to extract key information and concepts relevant to GVDs and regulatory submissions and generate structured content. This streamlines the literature review process and ensures that the latest evidence is incorporated into the documents.
Automation of Document Generation
AI-driven software can automate the generation of GVDs and regulatory submissions by integrating data analysis, literature review, and content generation capabilities. This reduces the time and resources required to create these documents while improving accuracy and consistency.
According to Henry Levy, President of Life Sciences at Clarivate,
“AI/ML is already used to pre-populate submissions to the authorities, for example, by pre-filling set fields” [10]. “GenAI could take this to another level with the ability to generate probably 95 percent of that content” [10]. “With proper oversight, GenAI has potential to drive efficiencies and cut the time needed for a regulatory submission”.“There is also scope for innovating new drugs” within the next decade [10].
Customization and Localization
AI allows tailoring GVDs to specific markets, considering regional guidelines, healthcare systems, and cultural differences. Localization ensures that GVDs resonate with local stakeholders.
Risk Assessment and Compliance
AI algorithms assess regulatory risks by analysing historical data and identifying potential issues. They help ensure compliance with regulatory requirements.
Predictive Analytics
AI algorithms can predict the potential impact of different pricing and market access strategies on the value of a product, helping companies make informed decisions during the development and commercialization process. This predictive analytics capability enhances the strategic planning and decision-making around GVDs and regulatory submissions.
Lastly, similar to techniques used in the financial industry as described earlier, dossier developers may look at the dossiers of similar health technologies which have received a positive reimbursement decision and extract “positive words” to include in their own dossiers as well as excluding “negative words” found in dossiers of technologies with a negative reimbursement decision [9]. Dossiers may be developed in a way that is “more friendly to machine parsing and processing” [3]. However, whether this strategy would be beneficial will depend on who develop the algorithms and what criteria are used for assessment [9]. Algorithms are open to bias, collusion and manipulation [3]. It is important that the algorithms that are used are accurate and free from bias, because there is a feedback loop where the outputs further inform algorithms and other analytics [1]. Bias can have implications for reimbursement and costs for patients and the health system [11].
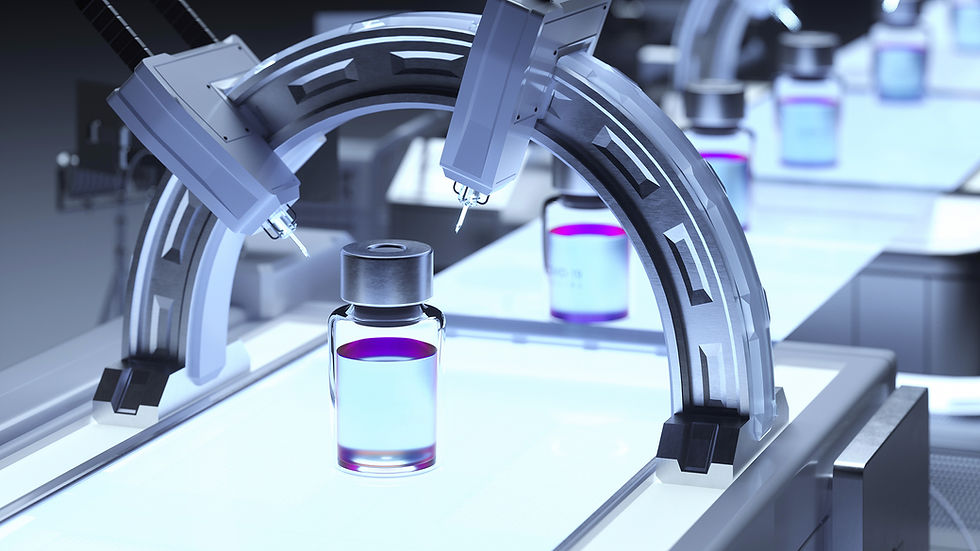
Therefore, the integration of AI in the creation of GVDs and regulatory submissions offers several benefits and challenges.
💫 Efficiency: AI automates time-consuming tasks such as data analysis and document generation, allowing teams to focus on higher-value activities.
💫 Accuracy: AI algorithms can analyse vast amounts of data with precision, reducing the risk of errors and ensuring the reliability of evidence presented in GVDs and regulatory submissions.
💫 Insights: AI-driven analytics provide valuable insights into market trends, payer preferences, and healthcare outcomes, enabling companies to tailor their value propositions and regulatory strategies accordingly.
💫 Speed to Market: By accelerating the document creation process, AI helps companies bring products to market faster, maximizing their potential for commercial success.
💫 Quality Assurance: While AI accelerates processes, human oversight is crucial to validate results and maintain accuracy.
💫 Ethical Use of Data: Privacy concerns and bias must be addressed when using patient data for AI-driven analyses.
💫 Interpretability: AI models should provide transparent explanations for their decisions to gain trust.
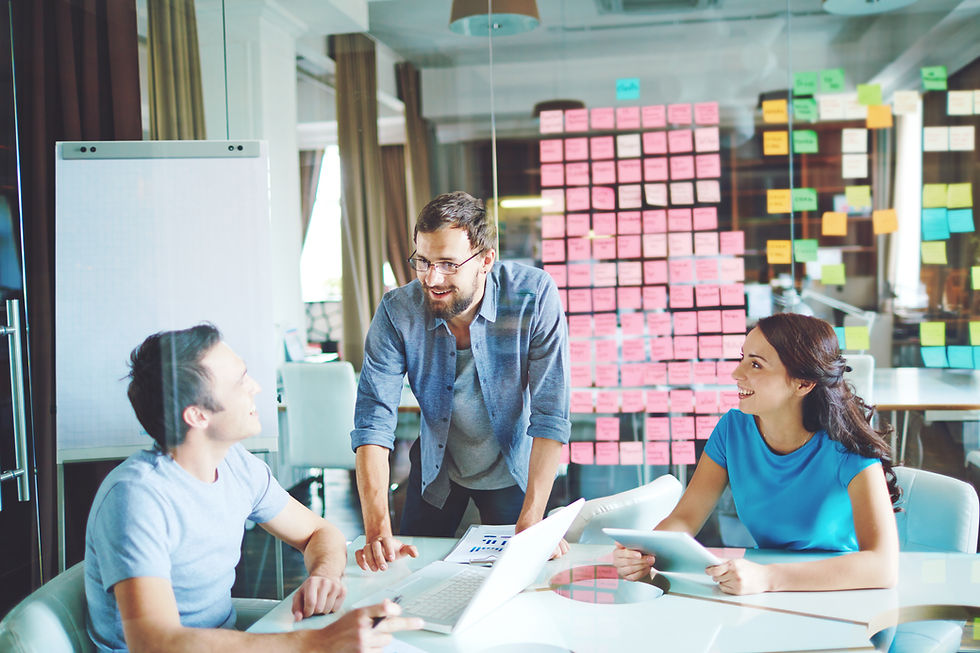
Conclusion
AI is revolutionizing the creation of GVDs and regulatory submissions for pharmaceutical and healthcare companies. By leveraging data analysis, NLP, automation, and predictive analytics, AI enables companies to craft compelling and evidence-based documents that support the value proposition of their products and facilitate regulatory approval. The integration of AI not only enhances efficiency and accuracy but also provides valuable insights that can drive strategic decision-making in the complex and dynamic healthcare landscape.
As the field evolves, collaboration between AI experts, healthcare professionals, and regulatory bodies will shape a more efficient and evidence-based future. AI’s role in shaping the future of regulatory submissions and GVDs will undoubtedly expand, offering new opportunities for innovation and advancement in the healthcare industry. Embracing AI-driven solutions is key to staying competitive and delivering value in the rapidly evolving healthcare ecosystem. However, while AI streamlines processes, human expertise remains indispensable in ensuring quality and ethical standards.
References
1. Naughton J. Companies are now writing reports tailored for AI readers – and it should worry us. The Guardian. Dec 5, 2020.
2. Shan Y. How AI Is Transforming Financial Reimbursement. Synced. https://medium.com/syncedreview/how-ai-is-transforming-financial-reimbursement-c5d8a1f13978. Published 2020. Updated May 16. Accessed May 26, 2021.
3. Cao S, Jiang W, Yang B, Zhang A. How to talk when a machine is listening: Corporate disclosure in the age of AI. Cambridge, MA: National Bureau of Economic Research;2020.
4. ICER. Guide to Understanding Health Technology Assessment (HTA). Institute for Clinical And Economic Review;2018.
5. Bujar M. Development of a Roadmap and Evaluation of Quality Decision-Making Practices during Medicines Development, Regulatory Review and Health Technology Assessment, University of Hertfordshire; 2018.
6. Khan S, Mordin M, Barrows S, et al. PNS169 SIMILARITIES & DIFFERENCES IN GLOBAL VALUE DOSSIER (GVD) AND ACADEMY OF MANAGED CARE PHARMACY (AMCP) DOSSIER DEVELOPMENT. Value in Health. 2019:S313.
7. Adeva JG, Atxa JP, Carrillo MU, Zengotitabengoa EA. Automatic text classification to support systematic reviews in medicine. Expert Systems with Applications. 2014;41(4):1498-1508.
8. Popoff E, Besada M, Jansen J, et al. Aligning text mining and machine learning algorithms with best practices for study selection in systematic literature reviews. Systematic Reviews. 2020;9:293.
9. Lock A. Implications of Digital Technologies for Healthcare and Human Behavior. Paper presented at: Digital Data-Driven Economy; June 28-29, 2021; Online.
10. Peachey C. GenAI in pharma: where are we today? European Pharmaceutical Review. https://www.europeanpharmaceuticalreview.com/article/190672/genai-in-pharma-where-are-we-today-artificial-intelligence-ai/. Published 2024. Updated 12 Jan 2024. Accessed 21 Feb, 2024.
11. Alami H, Lehoux P, Auclair Y, et al. Artificial Intelligence and Health Technology Assessment: Anticipating a New Level of Complexity. Journal of medical Internet research. 2020;22(7):e17707.
Comentarios